Exploring AI's Untapped Potential in Personalised Medicine
How AI is set to revolutionise patient-specific treatments by merging clinical and phenotypic data, driving a new era of targeted healthcare solutions
Add bookmark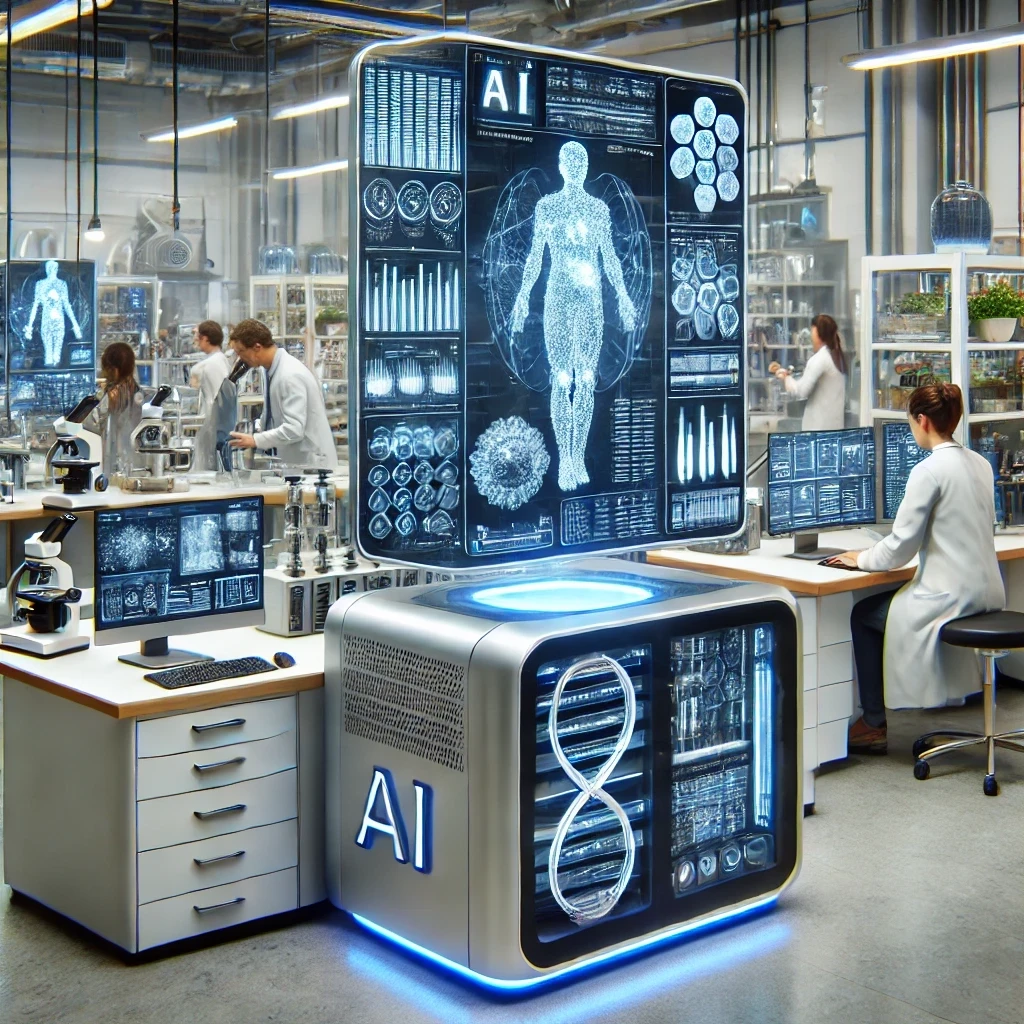
How AI is set to revolutionise patient-specific treatments by merging clinical and phenotypic data, driving a new era of targeted healthcare solutions: Insights from Dotmatics’ Alister Campbell
Artificial Intelligence (AI) has been making waves in the biopharma industry for its potential to revolutionise research and drug discovery. However, beyond the well-documented advantages in speeding up processes and enhancing data analysis, there is an underexplored realm where AI could truly change the game: personalised medicine. In this interview, Alister Campbell, Vice President and Global Head of Science & Technology at Dotmatics, delves into how AI is helping to bridge the gap between clinical data and early-stage research, allowing for more tailored treatments and transformative advancements in patient outcomes.
Pharma IQ: AI is transforming the biopharma industry in many ways. Could you begin by explaining how it’s impacting research and drug discovery?
Alister Campbell: AI has been part of research for years—though we used to call it computational chemistry! Today, AI is transforming several areas, including drug repurposing and predictive modelling. But what excites me most is its ability to merge clinical data with early-stage R&D data. This integration allows us to identify better druggable targets and discover key biomarkers. By combining vast datasets, AI helps us uncover insights that would have been impossible to identify before, leading to more efficient drug development and a faster route to personalised treatments.
AI’s Role in Targeting Personalised Therapies
Pharma IQ: Personalised medicine is an exciting area of advancement in healthcare. How is AI contributing to this field, and what improvements are you seeing in patient outcomes?
Alister Campbell: I believe AI has the greatest potential in personalised medicine, particularly in how it allows us to better understand the biology of individual patients. By using AI to combine clinical data with phenotypic and genotypic information, we can make much more accurate predictions about which treatments will work for specific patient groups. This is especially important in oncology, where every patient’s response to treatment can vary dramatically. AI allows us to tailor treatments more precisely, and that’s going to be game-changing for patient outcomes.
The Lab-in-the-Loop Concept: Real-World Data Meets AI
Pharma IQ: Could you explain more about the concept of integrating real-world data from clinical settings with AI models in R&D?
Alister Campbell: There’s a concept emerging called “lab-in-the-loop,” where data from clinical environments is constantly fed back into AI models. This real-world data, including phenotypic and clinical data from patients, is used to refine predictions and improve decision-making in R&D. For instance, we can identify cell populations or biomarkers that might predict how a patient will respond to treatment, helping us develop more effective therapies. In areas like oncology, this could be revolutionary, allowing us to create treatments that are as individual as the patients themselves.
AI’s Potential in Oncology and Target Identification
Pharma IQ: You’ve touched on the use of AI in oncology. Can you talk about how AI is enhancing target identification and the implications for drug development?
Alister Campbell: One of AI’s key roles in drug development is in target identification—finding the right molecules to go after in a patient’s biology. AI helps us identify these targets faster and with more accuracy by analysing vast datasets. This means we can design drugs that are more likely to succeed in clinical trials. With the amount of clinical and R&D data we can now access, AI can sift through millions of data points and highlight the most promising drug targets, which is critical for developing personalised therapies that work.
Overcoming the Challenges of Data Silos
Pharma IQ: What are some of the main challenges when integrating AI into biopharma workflows, particularly in the context of personalised medicine?
Alister Campbell: The main hurdle remains data silos—different departments or even entire organisations storing their data separately, which makes it difficult to use AI to its full potential. If we can’t access comprehensive datasets, the AI models can’t generate the best predictions. At Dotmatics, we’re working on solutions that bring together clinical data, cell-based assays, R&D results, and even phenotypic data in one centralised system. This makes the data more accessible and usable, allowing AI to do its job more effectively and helping us deliver on the promise of personalised medicine.
AI’s Role in Cost Reduction and Efficiency
Pharma IQ: Beyond personalised medicine, how is AI contributing to cost reduction and increased efficiency in drug development?
Alister Campbell: AI plays a huge role in driving down the costs of drug development. It can identify potential failures early in the development process, saving months or even years of work and millions in costs. Predictive modelling allows us to simulate how drugs will behave in the human body, which reduces the need for costly and time-consuming physical testing. This isn’t just good for the bottom line—it also means we can get life-saving treatments to patients faster.
AI and the Future of Personalised Medicine
Pharma IQ: Looking ahead, what do you think the future holds for AI in personalised medicine?
Alister Campbell: The future of AI in personalised medicine is incredibly exciting. I believe we’re just scratching the surface. As AI continues to improve, we’ll be able to make more accurate predictions about patient responses to treatments, and we’ll see real-world data playing an even bigger role in drug development. The ultimate goal is to deliver treatments that are tailor-made for each patient, improving outcomes and reducing the risk of ineffective therapies. Over the next few years, I expect AI to completely change the way we think about and approach personalised medicine, particularly in areas like oncology, where every patient is unique.
Conclusion:
As Alister highlights, the future of AI in personalised medicine is full of untapped potential. By integrating clinical data with R&D efforts, AI is paving the way for more targeted treatments that cater to the unique biological makeup of each patient. From enhancing target identification to overcoming data silos, AI is poised to transform healthcare, particularly in areas like oncology where the need for personalised therapies is most urgent. The next few years will be crucial in realising AI’s potential to deliver faster, more effective treatments that improve patient outcomes.
Further Reading
For more insights on how AI is maximising ROI in Drug Discovery and Development, download Pharma IQ's Report.
Find out how AI is Maximising Patient Recruitment and Retention in Clinical Trials with these Frequently Asked Questions.
Join Us to Learn More
Eager to dive deeper and learn how to overcome integration challenges from real-world case studies?
Join industry leaders and experts at the AI Pharma & Healthcare Event, where you can gain firsthand insights into successful AI integration strategies. This is your opportunity to learn from the successes and challenges faced by others and to network with professionals who are at the forefront of AI technology in the pharmaceutical industry.
📅Event Details
Event: AI Pharma & Healthcare Summit
Dates: 24-26 September 2024
Location: Amsterdam Marriott Hotel
Event Website: AI Pharma & Healthcare Summit