Realizing the promise of AI in biopharma
The world of biopharma could see huge benefits from embracing AI, but it will need to invest in data preparation and overcome an internal resistance to change
Add bookmark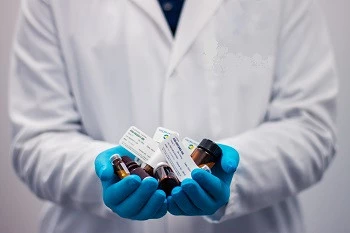
The development of new pharmaceuticals is a long and costly process, with less than 10% of clinical drug candidates reaching approval.
According to Ben Faircloth, Clay Heskett and Anne Dhulesia, Partners at L.E.K. Consulting, there is widespread recognition among biopharma companies that the R&D process is inefficient and that this inefficiency will keep growing if left unaddressed. This dynamic is partly driven by the increasingly complex nature of the biology underpinning the discovery of new molecules, increasing regulation and the fact that defining clinical trials is getting harder as patient populations need to be defined more precisely to show the desired effect.
AI has the potential to help curb this trend, promising innovative solutions that address drug development pain points:
- Improving the quality of candidates (e.g., improved target validation)
- Optimizing clinical trial design (e.g., biomarker based screening)
- Reducing time to complete activities (e.g., ADME1 parameter prediction)
- Reducing costs (e.g., enhanced use of in silico database-trained methods)
In addition to streamlining steps across the R&D process, AI solutions also have the potential to redefine the process itself by cutting out steps altogether.
Caution holds back adoption
Biopharma has been more cautious in adopting AI than other industries for a number of reasons.
First, there are unclear “real-world” benefits. Publicly documented use cases often fail to define the tangible impact on R&D cost, timelines or overall probability of success.
Second, interpreting AI insights can be challenging. Biopharma stakeholders often find it hard to understand the decision-making process underpinning an AI solution, resulting in a lack of buy-in to findings.
Third, the availability of relevant and readily usable biopharma proprietary data is limited, and it often takes significant time to clean, identify and extract the data required to enable AI.
Fourth, biopharma has an internal resistance to change, and the industry is known for its cautious nature. This is particularly true of R&D teams, which tend to be wary of new approaches given how much is at stake if things go wrong.
Finally, the AI landscape is evolving rapidly, making it challenging for biopharma to “pick winners”.
Lowering the barriers
Despite the barriers to deployment, biopharma’s interest in AI remains high. AI providers are increasingly targeting their solutions to address specific industry needs, matching biopharma expectations and building trust by providing more precise, comprehensible and substantiated evidence.
To accelerate the advance of AI, biopharma companies and AI providers can follow a few key principles to lower barriers to adoption.
Biopharma companies:
- Improve the collection and storage of internal data, and be ready to invest in data preparation.
- Facilitate data sharing across divisions, R&D projects and, potentially, beyond the organization.
- Have realistic expectations regarding what AI can provide.
- Lead a change management effort aimed at embracing AI. Communicate the value proposition of AI, offer specific training to consumers of AI (such as R&D staff) and adjust job roles as AI becomes integrated into a new R&D process.
AI providers:
- Secure sufficient biopharma expertise to develop AI solutions, and use it in customer outreach and sales to create common ground.
- Provide real-world evidence, explain the underlying AI processes and educate customers on how to interpret results. As possible, focus solutions on customers’ disease areas or target drug types.
- Work with high-quality data, secured through licenses/partnerships, and/or invest in cleaning it so that it can be used in AI.
- Be realistic about what can be achieved in this nascent space. Do not overpromise and fail to meet targets.
- Be patient, and do not interpret customer conservatism as a lack of interest. Biopharma has good reasons to move cautiously when adopting new technology.
The way ahead
After several years of experimentation and field testing, the authors of this article – Ben Faircloth, Clay Heskett and Anne Dhulesia – believe it is time for biopharma players to plan the implementation of AI solutions more broadly in drug development. With the right focus from both AI companies and biopharma, a significant proportion of R&D projects will have an AI component within the next five years.
1 ADME = absorption, distribution, metabolism and excretion